MBR maths modelling II − a view from our guest bloggers at Ghent University
Youri Amerlinck, Bram De Jaegher, Wouter Naessens and Ingmar Nopens
Guest bloggers Youri Amerlinck, Bram De Jaegher, Wouter Naessens and Ingmar Nopens from BIOMATH, Department of Mathematical Modelling, Statistics and Bioinformatics, Faculty of Bioscience Engineering, Ghent University respond to Simon’s last blog on MBR maths modelling. Visit a BIOMATH-perspective: http://www.biomath.ugent.be/
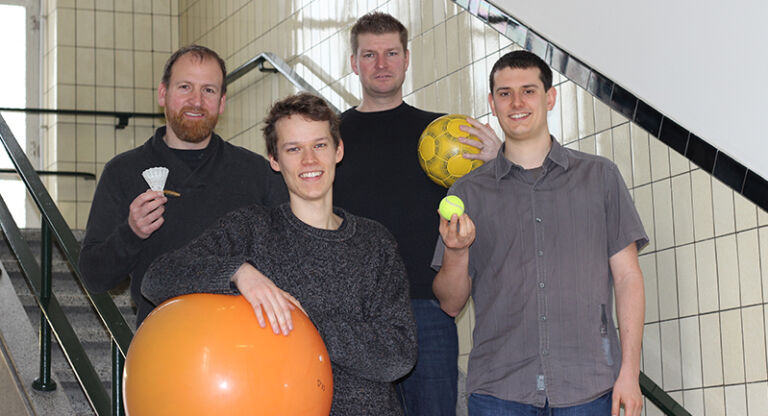
We have to admit that at first we were a bit sceptical, but in the end we enjoyed reading the blog post by Simon Judd about modelling and simulation of membrane bioreactors. We certainly embrace his message that practitioners and modellers should reach out to each other. With this in mind, it’s probably worth expanding on some of the points made
With infinite time and resources we can model just about anything. Unfortunately we only have limited amounts of these, which means that we sometimes have to assume (higher) spheres. But due to these limitations, as for any other project, matching the expectations and the potential is of utmost importance. Actually, one of the biggest pitfalls of a modelling project is overly enthusiastic stakeholders, making the expectations grow faster than the model’s and modeller’s potential.
That being said, the most important feature of a model is usually not the ultimate goal but the way towards that goal, i.e. the practical and theoretical understanding that comes along the modelling study. This is because of the very nature of a mathematical model is that it is a mere representation of what we know, and hence will never be ‘perfect’. This meticulous representation helps to remove the gut feeling from our analyses.
Confronting reality
The only way forward to improve models, i.e. to increase our knowledge, is to confront them with reality. This reality is captured in the experience, measurements and experiments available to the practitioners. However, to move forward and to increase our knowledge, we see a symbiotic relationship between the models and the experiments/measurements. Too many models have been published without any or with only limited confrontation with data. In this confrontation lies the chance to improve. Unfortunately, the confrontation with data also contains another pitfall. Some modellers have the tendency to fall in love with their model and (too) easily see 'a good fit' − given some imagination.
The ability to gather the necessary data to calibrate models, i.e. to match our thinking to reality, is in a lot of cases a cumbersome balancing act. The assumption of spheres has for decades been made in devices for measuring particle size distribution. A lot of (online) sensors apply (linear) correlations to link electrical signals with an actual measurable quantity (pH, dissolved oxygen, ammonia…). The sampling location may completely bias the measurement. And once we have enough confidence that the measurement is OK, the signal may start drifting, get interrupted and/or show other sensor failures. A lot of time is spent in identifying the correct data and filling in gaps to replace erroneous data.
The large investment in time for cleaning up data, especially for full-scale validation exercises, is a lot more challenging than at lab scale. Although often not appreciated by peer reviewers, making these valuable case studies very scarce in literature, the full-scale applications are crucial for technology deployment. Moreover, upscaling laboratory results is a scientific endeavour in itself.
In order to take decisions based on the experimental work and/or modelling work, the possibility and magnitude of errors, i.e. the inherent uncertainty, needs to be addressed. The nice thing is that a modelling framework offers an excellent environment to quantify this uncertainty and gives access to a platform to discuss.
Tackling the fouling problem
So, in order to tackle the fouling problem, practitioners and modellers are in some ways struggling with the same challenges. As Simon says, the multitude of fouling models around illustrates perfectly that our understanding of the process is far from complete. However, the world sometimes perceives this as if modelling work is unhelpful in battling the fouling problem.
The modellers, on the other hand, should test their models on real-life installations and not be scared if there happens to be a mismatch with the data, since this means extra knowledge can be gained − though this is often a tough sell. The only way forward is thus for practitioners and modellers to sit together and discuss − or even rewrite − the filtration theory with an open mind.
Finally, there is a point where we have to disagree with Simon. It is a big world indeed, and we certainly don’t advocate a flat world vision (as we are happy with spheres), but instead of the advice to wait and see, we propose to actually reach out and make a difference.